Certainly, data quality is a critical factor that can affect the success of any business. Whether it is for generating revenue, making informed decisions, or improving productivity, high-quality data is essential.
If low-quality data is used, it can cause businesses to miss out on potential opportunities, make poor decisions, and result in a negative return on investment. Therefore, to ensure the data is of high quality, people working closely with data must follow the data quality metrics and measures.
The good news is that by ensuring high-quality data, you can gain a competitive advantage and make better decisions to achieve your business objectives through putting metrics of quality data into practice.
Let’s learn everything about data quality metrics, their dimensions, and how to measure them. So let’s get started!
What are Data Quality Metrics?
Data quality defines if the data is accurate, complete, and reliable, and suitable for the use.
Data quality is evaluated using data quality metrics, which tells us how valuable and relevant the data is and if it can be trusted.
Evaluation is not enough the metrics of data quality help identify the difference between high-quality and low-quality data.
Significance of Data Quality Metrics
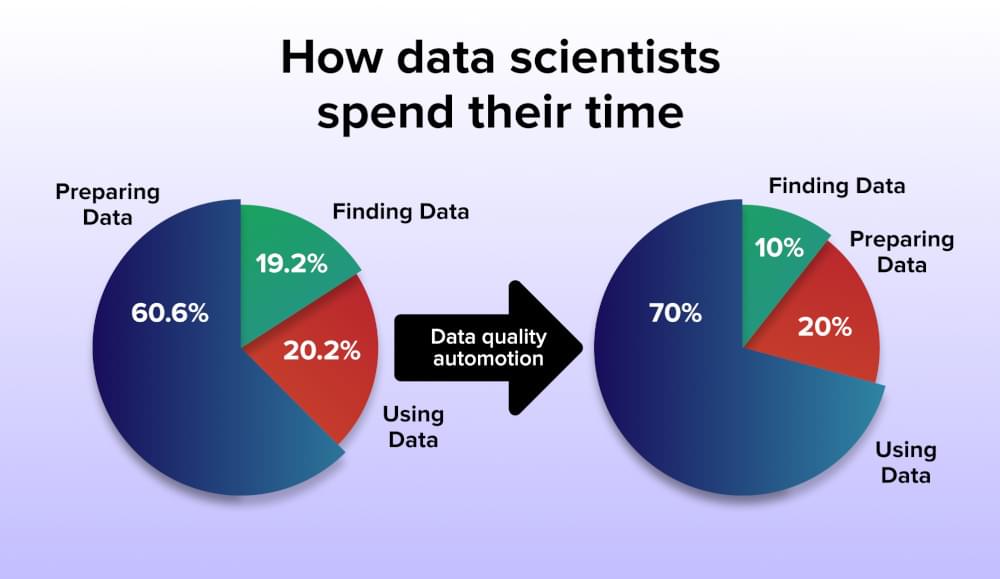
According to a survey, data cleaning and organization take up 60% of a data analyst’s workload, with 19% devoted to scraping. So basically, data analysts spend a whopping 80% of their time just getting the data ready for analysis this is mainly because preparing and managing quality data for analysis is a critical step that ensures accurate and reliable insights can be drawn from the data.
Data quality also helps organizations and businesses achieve various benefits, such as:
- Optimized decisions which improves the strategies
- Reduce risks
- Seize opportunities
- Comply with regulations and industry standards
- Optimized Profits
- Competitive advantage
- Increased user trust
Reliable and accurate data also leads to increased operational efficiency, reduced costs, and improved overall business performance. Furthermore, high-quality data is essential for providing better customer experiences by ensuring that customer information is up-to-date and accurate.
According to Forbes, 84% CEOs are concerned about the quality of the data on the basis of which they take decisions. As we have discussed previously, data of poor quality is problematic and creates poor business insights.
Let’s not forget that the better the quality of your data, the more benefits you can get from it.
Data Quality Dimensions and Categories
So far, we have gained a clear understanding of what data quality is and its significance. Now, let’s explore the data quality KPIs related to data quality to further enhance our understanding of the topic. According to studies, there are six dimensions of data quality, and we will explore each one in detail. Let’s dive in.
1. Accuracy
Accuracy tells us how accurate the data is, and if the values are correct and precise, and is measured using statistical methods. However, to ensure data accuracy, you must be very careful, as accuracy can be lost if the data is outdated, manually entered, or while transferring data.
When it comes to industries such as healthcare and finance, accuracy matters the most, even the slightest mistake can have severe consequences.
2. Completeness
Completeness refers to whether all of the required fields are complete in the data. If the data is incomplete, it would be considered useless. That’s why completeness is essential for data quality. While calculating the data, all the fields on record and attribute levels must be considered. To ensure that the data is complete, you must check the following:
- All required or mandatory fields, such as ‘Phone number’
- All optional fields, such as Interest Field
- All relevant and irrelevant fields for a particular record
3. Consistency
Consistency refers to the degree to which data is consistent with other data sources and within datasets. With the technological advancements, businesses store their valuable data across various sources and devices to avoid data loss.
Data consistency is achieved when the data is uniform across all of these sources. Therefore, to ensure data quality and consistency, it’s important to be watchful during data entry, data modifications, and data integration.
When the data is inconsistent, it can create significant problems. It may introduce errors and conflicts, which ultimately reduce the accuracy and reliability of the valuable data.
4. Timeliness
Timeliness refers to the degree to which data is available and updated in a timely manner. Yet, it’s essential to distinguish between data that is updated and data that is available. If the data is up-to-date but not available when needed, it can be considered poorly timed data.
This can have negative consequences for decision-making and the organization’s reputation. Therefore, it’s crucial to monitor and measure this data quality metric.
Moreover, timeliness holds significant importance in database management and evaluation, as it tells if the required information is available at the requested time. To properly evaluate timeliness, you must consider both the frequency of data availability and check if the data is updated.
5. Validity
Validity refers to the degree to which data matches established rules and standards. Invalid data can result from data entry errors due to different formats, data collected from unreliable sources, or data being stored or processed incorrectly. It is important to keep in mind that invalid data will ultimately affect the completeness of the data
6. Uniqueness
Uniqueness refers to the degree to which data values are unique and not repeated within datasets. This is a vital data quality metric that plays a significant role in achieving high-quality data by maintaining data accuracy in the records.
Sometimes, data can be repeated because it’s outdated or because lots of transfers are made. Even though it doesn’t happen a lot, we still need to pay attention to make sure we don’t have duplicate data.
Now let’s learn how we can measure the data quality metrics. We have made it super easy for you so, that you can quickly grab the data quality KPI’s and ways to measure them with ease. Cool right?
Also, see: Top 17 data analysis tools for businesses
Data Quality Metrics and measures
Dimension | Real World Definition | Measuring the dimension |
---|---|---|
Accuracy | How accurate the data is? | Use cross-validation techniques. Validate against trusted data |
Completeness | Is the data complete? | Compare the data with the sample data. Calculate the percentage of missing values |
Consistency | Is the data uniform across the other sources? | Map data between different sources. Integrate data into a single system |
Timeliness | Is the data available when you need it? | Calclulate time difference between data collection and data availability. Calclulate how often the data is updated. |
Validity | Is the data according to the set rules and regulations of the organization? | Calclulate percentage of invalid values. Validate the data in accordance to business rules and requirements. |
Uniqueness | Is the data unique across all of the sources? | Calclulate percentage of duplicate records. Set unique identifiers to identify duplicate records |
Data Quality Metrics in Action
When measuring data quality, it is essential to consider both the accuracy and quality of the data. To achieve high quality, check out these best practices for optimizing data quality.
1. Set your data quality metrics:
For every business, the business model varies, Therefore, you should determine the specific metrics according to your business objectives and goals for which you want to measure data quality. These metrics could include completeness, accuracy, consistency, timeliness, uniqueness, and validity. Overall, for businesses, these data quality metrics hold significant importance for:
- Top Management dealing with or presenting the data to clients
- Understanding where are the loopholes in the data to find accuracy
- Identifying incomplete, Invalid, and inconsistent data
2. Establish Data Quality rules:
Once you have identified your data quality metrics, set rules for each of them, such as how to measure them, what would be considered good quality data, etc, according to your business objectives.
Let’s say, if you have chosen completeness as one of your metrics, then you have to set a rule that all data fields must be completed to be considered valid.
When you’re dealing with data, it’s important to have rules in place to decide which data is useful and which needs to be changed, in simple words set the ranges that demonstrates the high and low quality data.
With the rapid advancement of technology, more and more data is being stored digitally rather than in traditional file formats and data quality has ultimately become more important. With so much data available, it’s crucial to make sure it’s accurate and reliable.
According to a study by Experian on Global Data Management, good quality data is extremely important if a company wants to do well in the digital world , gain competitive advantage, and be financially stable in the future. To accomplish this, data must be accurate and trustworthy, free of human errors. So, in order to establish uniformity throughout the firm, all entities involved in data management should accept the regulations.
3. Develop Data Quality Tests
Create tests that can be used to measure the data against the established rules. These tests could be automated or manual, depending on the complexity of the data and the rules.
4. Run Data Quality Tests
Once you have developed your tests, run them on the data to measure its quality. Record the results of each test. This is said to be the best way to build trust around data. The following checkpoints should be checked in the data to look for poor-quality data:
- Fields with no or little information
- Inaccurate and incomplete data
- Irregular Formatting
- Redundant items
- Old record that needs to be updated
Recording the results of each quality test is crucial for ensuring the reliability and accuracy of your data. It’s important to run regular quality checks to avoid overlooking errors, even small ones. This way, you can identify and correct any inaccuracies, ultimately improving the overall quality of your data.
5. Analyze the data quality results
After you run quality checks on your data, it’s important to analyze the results and see if your data meets the rules you have specified. This is a way to measure how good the quality of your data is. Furthermore, you can use this information to get valuable insights into why there are problems with the data quality and what you can do to fix them. With these insights, you can optimize the rules and make the data more valuable and reliable.
6. Improve data quality
Once you’ve identified areas where your data needs improvement, it’s important to make changes and take action to fix the problems. This might require changing the way you enter data, modifying rules for data quality metrics, or creating new ones.
You can also improve the quality of the data by implementing the changes needed to correct errors found in the data and making strategies to prevent the same mistakes from happening again in the future. By continuously making these changes, you can improve the overall quality of your data and make it more accurate and reliable.
If you think improving data quality is one time process the answer is NO! it’s a never-ending journey!
To maintain high-quality data, constant efforts and regular testing are a must. Reviewing data quality rules and regulations on a frequent basis is essential if you want the quality to improve constantly. The business environment is constantly changing, so managers need to come up with new data quality rules to optimize the quality of the data by making it reliable and accurate.
7. Monitor data quality:
It’s vital to constantly monitor your data to make sure it meets your defined rules for data quality. This gives you the flexibility to make any changes needed to maintain the high quality of your data. Regular monitoring is an essential data quality practice that ensures the reliability and accuracy of your data.
Web Scraping and Data Quality
Web scraping is the process of automatically collecting data from websites. It is widely used in various industries, such as market research, price monitoring, and data analysis.
Let’s see how web scraping is linked to data quality.
With top-notch scraping tools, you can get quality and relevant data from the most complex websites and on-demand too. The Crawlbase Crawling API is built using cutting-edge technology that ensures high-quality data collection.
The use of artificial intelligence and machine learning allows the scraper to adjust to website updates and gather the most updated data with ease. Furthermore, the Crawling API handles parsers, proxies, and browsers and automatically scrapes the web for you.
Frequently Asked Questions(FAQs)
1. What are the five metrics of quality data?
According to EHDI Data Quality Assessment, there are six data quality metrics, and each of them holds significant importance in measuring the quality of data.
- Accuracy tells how accurate the data is.
- Completeness defines if the data is complete.
- Consistency is to checks if the data uniform across the other sources.
- Timeliness tells if the data is available when you need it.
- Validity give insights if the data according to the set rules and regulations of the organization.
- Uniqueness is to check if the data unique across all of the sources.
2. How to measure data quality using a metric based approach?
In metric-based approach to measure data quality requires analyzing multiple aspects of the data to establish the data quality metrics. The steps for evaluating data quality using a metric-based method are as follows:
- Set your data quality metrics
- Establish Data Quality rules
- Develop data quality tests
- Run data quality tests
- Analyze the data quality results
- Improve data quality
- Monitor data quality
3. What are data quality metrics?
Data quality metrics are the KPI’s that are used to measure the quality of data. Data quality metrics help organizations and businesses improve data quality and ultimately make better strategies for their businesses’ growth.
Conclusion
There is no doubt that data is incredibly significant for every business since it is used to gather insights, opportunities, competitive advantages, and, most importantly, user trust. However, not all the data provides the same values, only the data which is of high quality. Therefore, organizations should pay serious attention to the quality of their data by implementing data quality metrics.
In this blog, we discussed the six data quality metrics and how to utilize them to boost data quality. We also discussed how to measure and monitor data quality and how to apply these metrics to practice effectively. We hope the knowledge we’ve provided has informed you of the value of data quality and its importance.